Complex Support System for Visually Impaired Individuals
DOI:
https://doi.org/10.58190/imiens.2022.1Keywords:
visually impaired, support system, object detection, deep learningAbstract
It is very difficult for visually impaired individuals to avoid obstacles, to notice or recognize obstacles in distance, to notice and follow the special paths made for them. They continue their lives by touching these situations or finding solutions with the help of a walking stick in their hands. Due to these safety problems, it is difficult for visually impaired individuals to move freely and these situations affect individuals negatively in terms of social and health. In order to find solutions to these problems, a support system has been proposed for visually impaired individuals. The vision support system includes an embedded system with a camera with an audio warning system so that the visually impaired individual can identify the objects in front of him, and a circuit with an ultrasonic sensor so that he can detect the obstacles in front of him early and take precautions. The object recognition system is realized with convolutional neural networks. The Faster R-CNN model was used and in addition to this, a model that we created, which can recognize 25 kinds of products, was used. With the help of the dataset we created and the network trained with this dataset, the visually impaired individual will be able to identify some market products. In addition to these, auxiliary elements were added to the walking sticks they used. This system consists of a camera system that enables the visually impaired individual to notice the lines made for the visually impaired in the environment, and a tracking circuit placed at the tip of the cane so that they can easily follow these lines and move more easily. Each system has been designed separately so that the warnings can be delivered to the visually impaired person quickly without delay. In this way, the error rate caused by the processing load has been tried to be reduced. The system we have created is designed to be wearable, easy to use and low-cost to be accessible to everyone.
Downloads
References
Islam, M.M., M.S. Sadi, K.Z. Zamli, and M.M. Ahmed, Developing walking assistants for visually impaired people: A review. IEEE Sensors Journal, 2019. 19(8): p. 2814-2828.
Kuriakose, B., R. Shrestha, and F.E. Sandnes, Tools and technologies for blind and visually impaired navigation support: a review. IETE Technical Review, 2022. 39(1): p. 3-18.
Simões, W.C., G.S. Machado, A. Sales, M.M. de Lucena, N. Jazdi, and V.F. de Lucena, A review of technologies and techniques for indoor navigation systems for the visually impaired. Sensors, 2020. 20(14): p. 3935.
Choi, J., S. Jung, D.G. Park, J. Choo, and N. Elmqvist. Visualizing for the non‐visual: Enabling the visually impaired to use visualization. in Computer Graphics Forum. 2019. Wiley Online Library.
Real, S. and A. Araujo, Navigation systems for the blind and visually impaired: Past work, challenges, and open problems. Sensors, 2019. 19(15): p. 3404.
Zhang, J., K. Yang, A. Constantinescu, K. Peng, K. Müller, and R. Stiefelhagen. Trans4Trans: Efficient transformer for transparent object segmentation to help visually impaired people navigate in the real world. in Proceedings of the IEEE/CVF International Conference on Computer Vision. 2021.
Tapu, R., B. Mocanu, and T. Zaharia, Wearable assistive devices for visually impaired: A state of the art survey. Pattern Recognition Letters, 2020. 137: p. 37-52.
Manjari, K., M. Verma, and G. Singal, A survey on assistive technology for visually impaired. Internet of Things, 2020. 11: p. 100188.
Aruna, M.A., M.B. Mol, M. Delcy, and P.D.M. ME, Rduino Powered Obstacles Avoidance For Visually Impaired Person. International Journal of Engineering and Information Systems (IJEAIS), 2018. 3(2).
Lin, T.-Y., M. Maire, S. Belongie, J. Hays, P. Perona, D. Ramanan, P. Dollár, and C.L. Zitnick. Microsoft coco: Common objects in context. in European conference on computer vision. 2014. Springer.
Li, Y., H. Huang, Q. Xie, L. Yao, and Q. Chen, Research on a surface defect detection algorithm based on MobileNet-SSD. Applied Sciences, 2018. 8(9): p. 1678.
Sai, B.K. and T. Sasikala. Object detection and count of objects in image using tensor flow object detection API. in 2019 International Conference on Smart Systems and Inventive Technology (ICSSIT). 2019. IEEE.
Taspinar, Y.S. and M. Selek, Object recognition with hybrid deep learning methods and testing on embedded systems. International Journal of Intelligent Systems and Applications in Engineering, 2020. 8(2): p. 71-77.
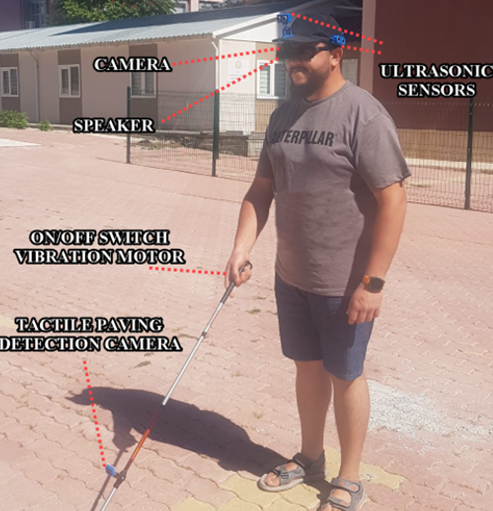
Published
Issue
Section
License
Copyright (c) 2022 Intelligent Methods In Engineering Sciences

This work is licensed under a Creative Commons Attribution-ShareAlike 4.0 International License.