Transfer Learning-Based Benchmarking Study for Diagnosis of COVID-19 from Lung CT Scans
DOI:
https://doi.org/10.58190/imiens.2022.4Keywords:
COVID-19, CT scan, transfer learning, deep learning, convolutional neural networksAbstract
The virus known as Severe Acute Respiratory Syndrome Coronavirus 2 (SARS-CoV-2) or Coronavirus Disease 2019 (COVID-19), which emerged from the city of Wuhan in the People’s Republic of China, has affected the whole world. This disease, which is categorized as an epidemic disease, continues to increase despite the various measures taken. It is aimed to reduce death and infected people rates with vaccination studies, inspection and early diagnosis. On the other hand, new types of coronavirus cases are emerging and people are kept under surveillance to prevent the spread of the virus. By keeping the infected people under quarantine, the transmission of the epidemic to more people is prevented. For this reason, early diagnosis kits and tests are vital. Today, various abnormalities are detected by specialists thanks to medical imaging tools. On the other hand, this process is performed on medical images using image processing techniques. Thanks to methods such as image classification, image segmentation, image quantification and various operations such as object detection, localization and quantitative analysis on the object are performed. In this study, it is aimed to detect COVID-19 on lung CT scan images with deep learning methods. CNN-based state-of-art deep learning models, which were pre-trained with millions of images and applied transfer learning method for a similar problem, were used in this study. This process was performed by choosing VGG19, ResNet152 and MobileNetV2 models and the results were compared. According to the performance criteria, validation accuracy of 93.53%, 95% and 87.28% was obtained from VGG19, ResNet152 and MobileNetV2 models, respectively. These results show that these models give good results for the detection of COVID-19 from lung CT scan images.
Downloads
References
“Coronavirus disease (COVID-19).” https://www.who.int/emergencies/diseases/novel-coronavirus-2019/question-and-answers-hub/q-a-detail/coronavirus-disease-covid-19 (accessed Sep. 02, 2021).
“Coronavirus disease (COVID-19): How is it transmitted?” https://www.who.int/news-room/q-a-detail/coronavirus-disease-covid-19-how-is-it-transmitted (accessed Sep. 02, 2021).
“WHO Coronavirus (COVID-19) Dashboard | WHO Coronavirus (COVID-19) Dashboard With Vaccination Data.” https://covid19.who.int/ (accessed Sep. 02, 2021).
“COVID-19 testing - Wikipedia.” https://en.wikipedia.org/wiki/COVID-19_testing (accessed Sep. 03, 2021).
“COVID-19 diagnostic testing: advantages and disadvantages.” https://www.myamericannurse.com/covid-19-diagnostic-testing/ (accessed Sep. 03, 2021).
T. Ai et al., “Correlation of Chest CT and RT-PCR Testing for Coronavirus Disease 2019 (COVID-19) in China: A Report of 1014 Cases,” Radiology, vol. 296, no. 2, pp. E32–E40, 2020, doi: 10.1148/radiol.2020200642.
O. Gozes, M. Frid, H. Greenspan, and D. Patrick, “Rapid AI Development Cycle for the Coronavirus ( COVID-19 ) Pandemic : Initial Results for Automated Detection & Patient Monitoring using Deep Learning CT Image Analysis Article Type : Authors : Summary Statement : Key Results : List of abbreviati,” arXiv:2003.05037, 2020, [Online]. Available: https://arxiv.org/ftp/arxiv/papers/2003/2003.05037.pdf.
A. Narin, C. Kaya, and Z. Pamuk, “Automatic detection of coronavirus disease (COVID-19) using X-ray images and deep convolutional neural networks,” Pattern Anal. Appl., vol. 24, no. 3, pp. 1207–1220, 2021, doi: 10.1007/s10044-021-00984-y.
F. Shan et al., “Lung infection quantification of COVID-19 in CT images with deep learning,” arxiv.org, Accessed: Sep. 03, 2021. [Online]. Available: https://arxiv.org/abs/2003.04655.
X. Chen, L. Yao, and Y. Zhang, “Residual Attention U-Net for Automated Multi-Class Segmentation of COVID-19 Chest CT Images,” vol. 14, no. 8, pp. 1–7, 2020, [Online]. Available: http://arxiv.org/abs/2004.05645.
K. Simonyan and A. Zisserman, “Very deep convolutional networks for large-scale image recognition,” 3rd Int. Conf. Learn. Represent. ICLR 2015 - Conf. Track Proc., pp. 1–14, 2015.
K. R. Avery et al., “Fatigue Behavior of Stainless Steel Sheet Specimens at Extremely High Temperatures,” SAE Int. J. Mater. Manuf., vol. 7, no. 3, pp. 1251–1258, 2014, doi: 10.4271/2014-01-0975.
T. F. Gonzalez, “Handbook of approximation algorithms and metaheuristics,” Handb. Approx. Algorithms Metaheuristics, pp. 1–1432, 2007, doi: 10.1201/9781420010749.
A. Khan, A. Sohail, U. Zahoora, and A. S. Qureshi, “A survey of the recent architectures of deep convolutional neural networks,” Artif. Intell. Rev., vol. 53, no. 8, pp. 5455–5516, 2020, doi: 10.1007/s10462-020-09825-6.
“SARS-COV-2 Ct-Scan Dataset | Kaggle.” https://www.kaggle.com/plameneduardo/sarscov2-ctscan-dataset (accessed Sep. 10, 2021).
“Otsu’s method - Wikipedia.” https://en.wikipedia.org/wiki/Otsu%27s_method (accessed Sep. 04, 2021).
“Data augmentation - Wikipedia.” https://en.wikipedia.org/wiki/Data_augmentation (accessed Sep. 04, 2021).
V. Sangeetha and K. J. R. Prasad, “Syntheses of novel derivatives of 2-acetylfuro[2,3-a]carbazoles, benzo[1,2-b]-1,4-thiazepino[2,3-a]carbazoles and 1-acetyloxycarbazole-2- carbaldehydes,” Indian J. Chem. - Sect. B Org. Med. Chem., vol. 45, no. 8, pp. 1951–1954, 2006, doi: 10.1002/chin.200650130.
M. Sandler, A. Howard, M. Zhu, A. Zhmoginov, and L. C. Chen, “MobileNetV2: Inverted Residuals and Linear Bottlenecks,” Proc. IEEE Comput. Soc. Conf. Comput. Vis. Pattern Recognit., pp. 4510–4520, 2018, doi: 10.1109/CVPR.2018.00474.
A. Voulodimos, N. Doulamis, A. Doulamis, and E. Protopapadakis, “Deep Learning for Computer Vision : A Brief Review,” vol. 2018, 2018.
K. Sabanci., M.F. Aslan., E. Ropelewska., M.F. Unlersen, A. Durdu. “A Novel Convolutional-Recurrent Hybrid Network for Sunn Pest–Damaged Wheat Grain Detection,” Food Anal. Methods pp. 1748–1760, 2022. https://doi.org/10.1007/s12161-022-02251-0
M. Koklu, M. F. Unlersen, I. A. Ozkan, M. F. Aslan, and K. Sabanci, “A CNN-SVM study based on selected deep features for grapevine leaves classification,” Measurement, vol. 188, p. 110425, 2022/01/01/ 2022,
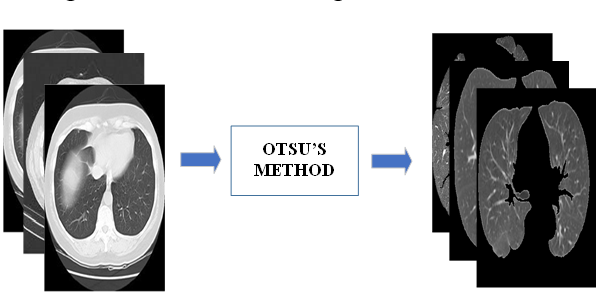
Downloads
Published
Issue
Section
License
Copyright (c) 2022 Intelligent Methods In Engineering Sciences

This work is licensed under a Creative Commons Attribution-ShareAlike 4.0 International License.