Classification of Hazelnut Species with Pre-Trained Deep Learning Models
DOI:
https://doi.org/10.58190/imiens.2023.14Keywords:
deep learning, hazelnut species, classification, pre-trained networkAbstract
A form of shelled nut in the Betulaceae family is the hazelnut. The majority of it is grown in Türkiye internationally. It grows in the provinces of Türkiye's Black Sea region, which is a significant global production hub. Hazelnuts can be eaten in a variety of ways and are a great source of protein, fat, fiber, vitamins, and minerals. There are numerous applications for hazelnuts in the food business. This study uses pre-trained networks to categorize eight of the most popular hazelnut kinds farmed in Türkiye. In this study, locally named hazelnut varieties grown in Türkiye were examined. An automated computer vision system was used to capture the images of the different hazelnut kinds. Our dataset includes a total of 2722 images, consisting of 155 palaz, 340 yagli, 399 deve disi, 236 tombul, 399 damat, 354 cakildak, 437 kara findik, and 402 sivri hazelnuts. Using transfer learning, the DenseNet121 and InceptionV3 models of convolutional neural networks were employed to categorize these images. The dataset was split into training and testing portions, respectively. With InceptionV3 and DenseNet121, respectively, the research revealed classification accuracy of 96.99% and 96.18%.
Downloads
References
A. İslam, "Hazelnut culture in Turkey," Akademik Ziraat Dergisi, vol. 7, no. 2, pp. 259-266, 2018, doi: doi.org/10.29278/azd.476665.
A. İslam, "Clonal selection in ‘Uzunmusa’ hazelnut," Plant Breeding, doi.org/10.1046/j.1439-0523.2003.00853.x vol. 122, no. 4, pp. 368-371, 2003/08/01 2003, doi: doi.org/10.1046/j.1439-0523.2003.00853.x.
A. Islam and A. I. Ozgüven, "Clonal selection in the Turkish hazelnut cultivars grown in Ordu province," in V International Congress on Hazelnut 556, 2000, pp. 203-208, doi: 10.17660/ActaHortic.2001.556.29.
J. S. Amaral, S. Casal, I. Citová, A. Santos, R. M. Seabra, and B. P. P. Oliveira, "Characterization of several hazelnut (Corylus avellana L.) cultivars based in chemical, fatty acid and sterol composition," European Food Research and Technology, vol. 222, no. 3, pp. 274-280, 2006/02/01 2006, doi: 10.1007/s00217-005-0068-0.
M. Yalçın and A. Arol, "Altın metalürjisi için yerli kaynaklardan aktif karbon üretimi," Türkiye XIII Madencilik Kongresi, vol. 413, p. 426, 1993.
Y. Unal, Y. S. Taspinar, I. Cinar, R. Kursun, and M. Koklu, "Application of Pre-Trained Deep Convolutional Neural Networks for Coffee Beans Species Detection," Food Analytical Methods, vol. 15, no. 12, pp. 3232-3243, 2022/12/01 2022, doi: 10.1007/s12161-022-02362-8.
M. Koklu, M. F. Unlersen, I. A. Ozkan, M. F. Aslan, and K. Sabanci, "A CNN-SVM study based on selected deep features for grapevine leaves classification," Measurement, vol. 188, p. 110425, 2022/01/01/ 2022, doi: doi.org/10.1016/j.measurement.2021.110425.
K. P. Ferentinos, "Deep learning models for plant disease detection and diagnosis," Computers and Electronics in Agriculture, vol. 145, pp. 311-318, 2018/02/01/ 2018, doi: doi.org/10.1016/j.compag.2018.01.009.
M. Dogan, Y. S. Taspinar, I. Cinar, R. Kursun, I. A. Ozkan, and M. Koklu, "Dry bean cultivars classification using deep cnn features and salp swarm algorithm based extreme learning machine," Computers and Electronics in Agriculture, vol. 204, p. 107575, 2023/01/01/ 2023, doi: doi.org/10.1016/j.compag.2022.107575.
Z. Ünal and H. Aktaş, "Classification of hazelnut kernels with deep learning," Postharvest Biology and Technology, vol. 197, p. 112225, 2023/03/01/ 2023, doi: doi.org/10.1016/j.postharvbio.2022.112225.
C. Koc, D. Gerdan, M. B. EmİNoĞLu, U. YegÜL, B. Koc, and M. VatandaŞ, "Classification of hazelnut cultivars: comparison of DL4J and ensemble learning algorithms," Notulae Botanicae Horti Agrobotanici Cluj-Napoca, vol. 48, no. 4, pp. 2316-2327, 12/22 2020, doi: 10.15835/nbha48412041.
A. Giraudo, R. Calvini, G. Orlandi, A. Ulrici, F. Geobaldo, and F. Savorani, "Development of an automated method for the identification of defective hazelnuts based on RGB image analysis and colourgrams," Food Control, vol. 94, pp. 233-240, 2018/12/01/ 2018, doi: doi.org/10.1016/j.foodcont.2018.07.018.
S. Bayrakdar, B. Çomak, D. Başol, and Y. İ, "Determination of type and quality of hazelnut using image processing techniques," in 2015 23nd Signal Processing and Communications Applications Conference (SIU), 16-19 May 2015 2015, pp. 616-619, doi: 10.1109/SIU.2015.7129899.
S. Solak and U. Altınışık, "Detection and classification of hazelnut fruit by using image processing techniques and clustering methods," Sakarya University Journal of Science, vol. 22, no. 1, pp. 56-65, 2018, doi: doi.org/10.16984/saufenbilder.303850.
S. A. Guvenc, F. A. Senel, and B. Cetisli, "Classification of processed hazelnuts with computer vision," in 2015 23nd Signal Processing and Communications Applications Conference (SIU), 16-19 May 2015 2015, pp. 1362-1365, doi: 10.1109/SIU.2015.7130094.
O. Keles and A. Taner, "Classification of hazelnut varieties by using artificial neural network and discriminant analysis," Spanish Journal of Agricultural Research, vol. 19, no. 4, pp. e0211-e0211, 2021, doi: doi.org/10.5424/sjar/2021194-18056.
I. Khosa and E. Pasero, "Feature extraction in X-ray images for hazelnuts classification," in 2014 International Joint Conference on Neural Networks (IJCNN), 6-11 July 2014 2014, pp. 2354-2360, doi: 10.1109/IJCNN.2014.6889661.
S. K. S. Al-Doori, Y. S. Taspinar, and M. Koklu, "Distracted Driving Detection with Machine Learning Methods by CNN Based Feature Extraction," International Journal of Applied Mathematics Electronics and Computers, vol. 9, no. 4, pp. 116-121, 2021, doi: doi.org/10.18100/ijamec.1035749.
M. Koklu and Y. S. Taspinar, "Determining the Extinguishing Status of Fuel Flames With Sound Wave by Machine Learning Methods," IEEE Access, vol. 9, pp. 86207-86216, 2021, doi: 10.1109/ACCESS.2021.3088612.
M. Koklu and K. Sabanci, "Estimation of credit card customers payment status by using kNN and MLP," International Journal of Intelligent Systems and Applications in Engineering, vol. 4, no. Special Issue-1, pp. 249-251, 2016.
M. Koklu, I. Cinar, and Y. S. Taspinar, "Classification of rice varieties with deep learning methods," Computers and Electronics in Agriculture, vol. 187, p. 106285, 2021/08/01/ 2021, doi: doi.org/10.1016/j.compag.2021.106285.
E. T. Yasin, I. A. Ozkan, and M. Koklu, "Detection of fish freshness using artificial intelligence methods," European Food Research and Technology, 2023/04/27 2023, doi: 10.1007/s00217-023-04271-4.
I. Cinar, Y. S. Taspinar, R. Kursun, and M. Koklu, "Identification of Corneal Ulcers with Pre- Trained AlexNet Based on Transfer Learning," in 2022 11th Mediterranean Conference on Embedded Computing (MECO), 7-10 June 2022 2022, pp. 1-4, doi: 10.1109/MECO55406.2022.9797218.
J. Huang et al., "Speed/accuracy trade-offs for modern convolutional object detectors," in Proceedings of the IEEE conference on computer vision and pattern recognition, 2017, pp. 7310-7311.
D. Singh et al., "Classification and Analysis of Pistachio Species with Pre-Trained Deep Learning Models," Electronics, vol. 11, no. 7, doi: 10.3390/electronics11070981.
C. Shorten and T. M. Khoshgoftaar, "A survey on image data augmentation for deep learning," Journal of big data, vol. 6, no. 1, pp. 1-48, 2019, doi: doi.org/10.1186/s40537-019-0197-0.
X. Zhang, X. Chen, W. Sun, and X. He, "Vehicle Re-Identification Model Based on Optimized DenseNet121 with Joint Loss," Computers, Materials & Continua, vol. 67, no. 3, 2021, doi: 10.32604/cmc.2021.016560.
E. T. Hastuti, A. Bustamam, P. Anki, R. Amalia, and A. Salma, "Performance of True Transfer Learning using CNN DenseNet121 for COVID-19 Detection from Chest X-Ray Images," in 2021 IEEE International Conference on Health, Instrumentation & Measurement, and Natural Sciences (InHeNce), 14-16 July 2021 2021, pp. 1-5, doi: 10.1109/InHeNce52833.2021.9537261.
C. Szegedy, V. Vanhoucke, S. Ioffe, J. Shlens, and Z. Wojna, "Rethinking the inception architecture for computer vision," in Proceedings of the IEEE conference on computer vision and pattern recognition, 2016, pp. 2818-2826.
O. Russakovsky et al., "ImageNet Large Scale Visual Recognition Challenge," International Journal of Computer Vision, vol. 115, no. 3, pp. 211-252, 2015/12/01 2015, doi: 10.1007/s11263-015-0816-y.
Y. S. Taspinar, "Light weight convolutional neural network and low-dimensional images transformation approach for classification of thermal images," Case Studies in Thermal Engineering, vol. 41, p. 102670, 2023/01/01/ 2023, doi: doi.org/10.1016/j.csite.2022.102670.
Y. S. Taspinar, M. Dogan, I. Cinar, R. Kursun, I. A. Ozkan, and M. Koklu, "Computer vision classification of dry beans (Phaseolus vulgaris L.) based on deep transfer learning techniques," European Food Research and Technology, vol. 248, no. 11, pp. 2707-2725, 2022/11/01 2022, doi: 10.1007/s00217-022-04080-1.
B. Kishore et al., "Computer-Aided Multiclass Classification of Corn from Corn Images Integrating Deep Feature Extraction," Computational Intelligence and Neuroscience, vol. 2022, p. 2062944, 2022/08/10 2022, doi: 10.1155/2022/2062944.
R. Butuner, I. Cinar, Y. S. Taspinar, R. Kursun, M. H. Calp, and M. Koklu, "Classification of deep image features of lentil varieties with machine learning techniques," European Food Research and Technology, vol. 249, no. 5, pp. 1303-1316, 2023/05/01 2023, doi: 10.1007/s00217-023-04214-z.
K. Tutuncu, I. Cinar, R. Kursun, and M. Koklu, "Edible and Poisonous Mushrooms Classification by Machine Learning Algorithms," in 2022 11th Mediterranean Conference on Embedded Computing (MECO), 7-10 June 2022 2022, pp. 1-4, doi: 10.1109/MECO55406.2022.9797212.
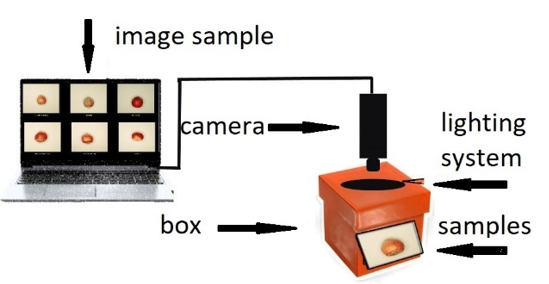
Downloads
Published
Issue
Section
License
Copyright (c) 2023 Intelligent Methods In Engineering Sciences

This work is licensed under a Creative Commons Attribution-ShareAlike 4.0 International License.