Realising Quality Control of Metal Products Used in Industry Through Deep Learning
DOI:
https://doi.org/10.58190/imiens.2024.114Keywords:
CNN, Defect and Defection, Metal Surface, Defect, Quality ControlAbstract
This study was carried out with the aim of detecting the defects on the surfaces of metallic products, which are frequently encountered in our daily lives and widely used in industry, with deep learning. Many metal products in the industry undergo different processes during the production phase. As a result of these processes, the detection of defects such as breakage, cracking, etc. on the surfaces of metal products is carried out by quality control personnel or production personnel. However, this error detection made by manpower both slows down production and causes overlooked errors. In order to easily detect these defects, in our study, we used a dataset consisting of 285 images in total with images of two different surface defects and images containing flawless metal parts and a CNN architecture, ResNet50 architecture, to defects. There are three classes in total in the dataset. Two of these classes consist of images of different types of defects on the surface of the metal piston part used in air conditioners, and one of them consists of images of perfect metal piston parts. Convolutional Neural Network (CNN) method was used to determine the features of the images. Precision, recall, F1-Score and accuracy metrics were used to measure the performance of the model. With the ResNet50 architecture, defects on the surfaces of metal piston parts are detected quickly and with high accuracy. As a result of the study, it was suggested that the proposed model can detect surface defects that occur in the usage areas of metal products in various sectors more quickly and accurately using deep learning. This shows that the quality control problems experienced in the industry can be reduced by using deep learning, saving time and manpower.
Downloads
References
[1] Wang, C. and H. Xie, MeDERT: A metal surface defect detection model. IEEE Access, 2023. 11: p. 35469-35478.
[2] Tao, X., et al., Automatic metallic surface defect detection and recognition with convolutional neural networks. Applied Sciences, 2018. 8(9): p. 1575.
[3] Lal, R., B.K. Bolla, and E. Sabeesh, Efficient neural net approaches in metal casting defect detection. Procedia Computer Science, 2023. 218: p. 1958-1967.
[4] Silenzi, A., et al., Ǫuality Control of Carbon Look Components via Surface Defect Classification with Deep Neural Networks. Sensors, 2023. 23(17): p. 7607.
[5] Neuhauser, F.M., G. Bachmann, and P. Hora, Surface defect classification and detection on extruded aluminium profiles using convolutional neural networks. International Journal of Material Forming, 2020. 13(4): p. 591-603.
[6] Konovalenko, I., et al., Steel surface defect classification using deep residual neural network. Metals, 2020. 10(6): p. 846.
[7] Wang, S., et al., Automatic detection and classification of steel surface defect using deep convolutional neural networks. Metals, 2021. 11(3): p. 388.
[8] Adibhatla, V.A., et al., Defect detection in printed circuit boards using you-only- look-once convolutional neural networks. Electronics, 2020. G(9): p. 1547.
[9] Zhao, H., Z. Yang, and J. Li. Detection of metal surface defects based on on YOLOv4 algorithm. in Journal of Physics: Conference Series. 2021. IOP Publishing.
[10] Su, Y., et al., A cascaded combination method for defect detection of metal gear end-face. Journal of Manufacturing Systems, 2022. 63: p. 439-453.
[11] Noroozi, M., et al., Towards Optimal Defect Detection in Assembled Printed Circuit Boards Under Adverse Conditions. IEEE Access, 2023.
[12] Baumgartl, H., et al., A deep learning-based model for defect detection in laser- powder bed fusion using in-situ thermographic monitoring. Progress in Additive Manufacturing, 2020. 5(3): p. 277-285.
[13] Gui, Y., et al., Detection, classification and prediction of internal defects from surface morphology data of metal parts fabricated by powder bed fusion type additive manufacturing using an electron beam. Additive Manufacturing, 2022. 54: p. 102736.
[14] Fang, X., et al., Research progress of automated visual surface defect detection for industrial metal planar materials. Sensors, 2020. 20(18): p. 5136.
[15] Liu, J., et al., Defect detection for metal base of TO-Can packaged laser diode based on improved YOLO algorithm. Electronics, 2022. 11(10): p. 1561.
[16] Abagiu, M.M., et al., Detecting Machining Defects inside Engine Piston Chamber with Computer Vision and Machine Learning. Sensors, 2023. 23(2): p. 785.
[17] Wang, J., et al., The quality inspection method of piston compressor assisted with the XGBOD model. International Journal of Refrigeration, 2023. 150: p. 158- 169.
[18] Chaudhari, C.V., Steel surface defect detection using glcm, gabor wavelet, hog, and random forest classifier. Turkish Journal of Computer and Mathematics Education (TURCOMAT), 2021. 12(12): p. 263-273.
[19] Mechanic Component Images (Normal / Defected) Available online at: https://www.kaggle.com/datasets/satishpaladi11/mechanic-component-images-no rmal-defected?select=Defected1
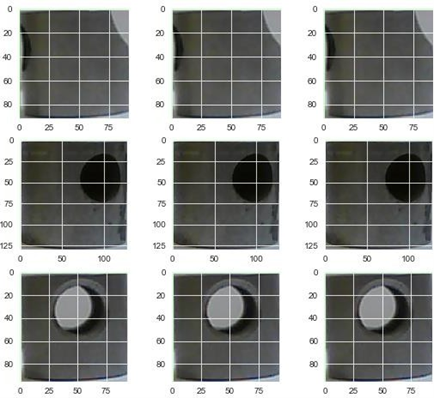
Downloads
Published
Issue
Section
License
Copyright (c) 2024 Intelligent Methods In Engineering Sciences

This work is licensed under a Creative Commons Attribution-ShareAlike 4.0 International License.