Real-Time Emotion Recognition Using Deep Learning Methods: Systematic Review
DOI:
https://doi.org/10.58190/imiens.2023.7Abstract
The seven basic facial expressions are the most important indicator of a person's psychological state, regardless of gender, age, culture, or nationality. These expressions are an involuntary reaction that shows up on the face for a short time. They show how the person is feeling—sad, happy, angry, scared, disgusted, surprised, or neutral. The visual system and brain automatically detect a person's emotion through facial expressions. Most computer vision researchers struggle to automate facial expression recognition. Human emotion-detection pioneers have also tried to mimic human automatic detection. Thus, deep learning techniques are the closest to mimicking human intelligence. Despite deep learning techniques, creating a system that can accurately distinguish between facial expressions is still difficult due to the diversity of faces and the convergence of some expressions that express different emotions. This systematic review presents a scientifically rich paper on deep learning-based facial expression emotion detection methods. From 2019 to the present, PRISMA was used to search and select research on real-time emotions. The study collected datasets from the mentioned period that were used to train, test, and verify the models presented in the relevant studies. Each dataset was fully explained in terms of number of items, type of data, etc. The study also compared relevant studies and identified the best technique. Furthermore, challenges to systems that detect emotions through facial expressions have been identified.
Downloads
References
B. G. K. Reddy, P. Yashwanthsaai, A. R. Raja, A. Jagarlamudi, N. Leeladhar, and T. T. Kumar, “Emotion Recognition Based on Convolutional Neural Network ( CNN ),” in International Conference on Advancements in Electrical, Electronics, Communication, Computing and Automation, 2021, pp. 1–5, doi: 10.1109/ICAECA52838.2021.9675688.
A. Sharma, K. Sharma, and A. Kumar, “Real-time emotional health detection using fine-tuned transfer networks with multimodal fusion,” Neural Comput. Appl., vol. 2, 2022.
M. K. Chowdary, T. N. Nguyen, and D. J. Hemanth, “Deep learning-based facial emotion recognition for human–computer interaction applications,” Neural Comput. Appl., vol. 8, 2021, doi: 10.1007/s00521-021-06012-8.
K. Wang, Y. Ho, Y. Huang, and W. Fang, “Design of Intelligent EEG System for Human Emotion Recognition with Convolutional Neural Network,” in IEEE International Conference on Artificial Intelligence Circuits and Systems (AICAS), 2019, pp. 142–145.
W. Liu, J. Qiu, W. Zheng, and B. Lu, “Comparing Recognition Performance and Robustness of Multimodal Deep Learning Models for Multimodal Emotion Recognition,” IEEE Trans. Cogn. Dev. Syst., vol. 14, no. 2, pp. 715–729, 2022.
L. Sandra, Y. Heryadi, Lukas, W. Suparta, and A. Wibowo, “Deep Learning Based Facial Emotion Recognition using Multiple Layers Model,” in International Conference on Advanced Mechatronics, Intelligent Manufacture and Industrial Automation, 2021, pp. 137–142, doi: 10.1109/ICAMIMIA54022.2021.9809908.
A. Landowska et al., “Automatic Emotion Recognition in Children with Autism: A Systematic Literature Review,” Sensors, vol. 22, no. 4, pp. 1–30, 2022, doi: 10.3390/s22041649.
S. Al-asbaily and K. Bozed, “Facial Emotion Recognition Based on Deep Learning,” in IEEE 2nd International Maghreb Meeting of the Conference on Sciences and Techniques of Automatic Control and Computer Engineering (MI-STA), 2022, vol. 22, no. 16, pp. 534–538, doi: 10.3390/s22166105.
S. Kaur and N. Kulkarni, “A Deep Learning Technique for Emotion Recognition Using Face and Voice Features,” in IEEE Pune Section International Conference, PuneCon 2021, 2021, pp. 1–6, doi: 10.1109/PuneCon52575.2021.9686510.
M. U. Khan, M. A. Abbasi, Z. Saeed, M. Asif, A. Raza, and U. Urooj, “Deep learning based Intelligent Emotion Recognition and Classification System,” in Proceedings - International Conference on Frontiers of Information Technology, FIT, 2021, pp. 25–30, doi: 10.1109/FIT53504.2021.00015.
A. I. Siam, N. F. Soliman, A. D. Algarni, F. E. Abd El-Samie, and A. Sedik, “Deploying Machine Learning Techniques for Human Emotion Detection,” Comput. Intell. Neurosci., 2022, doi: 10.1155/2022/8032673.
S. Palaniswamy and Suchitra, “A Robust Pose Illumination Invariant Emotion Recognition from Facial Images using Deep Learning for Human-Machine Interface,” 2019, doi: 10.1109/CSITSS47250.2019.9031055.
S. Yuvaraj, J. V. Franklin, V. S. Prakash, and A. Anandaraj, “An Adaptive Deep Belief Feature Learning Model for Cognitive Emotion Recognition,” 8th Int. Conf. Adv. Comput. Commun. Syst. ICACCS 2022, vol. 1, pp. 1844–1848, 2022, doi: 10.1109/ICACCS54159.2022.9785267.
G. Chartrand et al., “Deep learning: A primer for radiologists,” Radiographics, vol. 37, no. 7, pp. 2113–2131, 2017, doi: 10.1148/rg.2017170077.
L. Alzubaidi et al., “Review of deep learning: concepts, CNN architectures, challenges, applications, future directions,” J. Big Data, vol. 8, no. 1, 2021, doi: 10.1186/s40537-021-00444-8.
L. Arnold, S. Rebecchi, S. Chevallier, and H. Paugam-Moisy, “An introduction to deep learning,” Eur. Symp. Artif. Neural Networks, pp. 477–488, 2021, doi: 10.1201/9780429096280-14.
D. Canedo and A. J. R. Neves, “Facial expression recognition using computer vision: A systematic review,” Appl. Sci., vol. 9, no. 21, pp. 1–31, 2019, doi: 10.3390/app9214678.
A. Saravanan, G. Perichetla, and D. K.S.Gayathri, “Facial Emotion Recognition using Convolutional Neural Networks,” arXiv, vol. 1, 2019.
S. Albawi, T. A. Mohammed, and S. Al-Zawi, “Understanding of a convolutional neural network,” in International Conference on Engineering and Technology, ICET, 2018, pp. 1–6, doi: 10.1109/ICEngTechnol.2017.8308186.
X. Sun, P. Xia, L. Zhang, and L. Shao, “A ROI-guided deep architecture for robust facial expressions recognition,” Inf. Sci. (Ny)., vol. 522, pp. 35–48, 2020, doi: 10.1016/j.ins.2020.02.047.
R. Chauhan, K. K. Ghanshala, and R. C. Joshi, “Convolutional Neural Network (CNN) for Image Detection and Recognition,” in International Conference on Secure Cyber Computing and Communications, 2018, pp. 278–282, doi: 10.1109/ICSCCC.2018.8703316.
P. Giannopoulos, I. Perikos, and I. Hatzilygeroudis, “Deep learning approaches for facial emotion recognition: A case study on FER-2013,” Smart Innov. Syst. Technol., vol. 85, pp. 1–16, 2018, doi: 10.1007/978-3-319-66790-4_1.
S. H. Lee, “Facial data visualization for improved deep learning based emotion recognition,” J. Inf. Sci. Theory Pract., vol. 7, no. 2, pp. 32–39, 2019, doi: 10.1633/JISTaP.2019.7.2.3.
A. Y. Nawaf and W. M. Jasim, “Human emotion identification based on features extracted using CNN Human Emotion Identification Based on Features Extracted Using CNN,” in AIP Conference Proceedings, 2022, vol. 020010.
K. O’Shea and R. Nash, “An Introduction to Convolutional Neural Networks,” arXiv, pp. 1–11, 2015.
J. Gu et al., “Recent advances in convolutional neural networks,” Pattern Recognit., vol. 77, pp. 354–377, 2018, doi: 10.1016/j.patcog.2017.10.013.
C. Nwankpa, W. Ijomah, A. Gachagan, and S. Marshall, “Activation Functions: Comparison of trends in Practice and Research for Deep Learning,” arXiv, pp. 1–20, 2018.
J. T. Barron, “A general and adaptive robust loss function,” Proc. IEEE Comput. Soc. Conf. Comput. Vis. Pattern Recognit., vol. 2019-June, pp. 4326–4334, 2019, doi: 10.1109/CVPR.2019.00446.
C. Wan, D. Chen, Z. Huang, and X. Luo, “A wearable head mounted display bio-signals pad system for emotion recognition,” Sensors, vol. 22, no. 1, pp. 1–20, 2022, doi: 10.3390/s22010142.
H. D. Nguyen, S. H. Kim, G. S. Lee, H. J. Yang, I. S. Na, and S. H. Kim, “Facial Expression Recognition Using a Temporal Ensemble of Multi-Level Convolutional Neural Networks,” IEEE Trans. Affect. Comput., vol. 13, no. 1, pp. 226–237, 2022, doi: 10.1109/TAFFC.2019.2946540.
M. M. L. Joshi and M. S. Agarwal, “FACIAL EMOTION RECOGNITION USING DEEP LEARNING: A SURVEY,” OORJA, vol. 19, 2021.
R. Gill and J. Singh, “A Deep Learning Approach for Real Time Facial Emotion Recognition,” in 10th International Conference on System Modeling and Advancement in Research Trends, SMART, 2021, pp. 497–501, doi: 10.1109/SMART52563.2021.9676202.
J. C. Hung, K. C. Lin, and N. X. Lai, “Recognizing learning emotion based on convolutional neural networks and transfer learning,” Appl. Soft Comput. J., vol. 84, 2019, doi: 10.1016/j.asoc.2019.105724.
S. Norouzi and M. Ebrahimi, “A Survey on Proposed Methods to Address Adam Optimizer Deficiencies,” 2019.
A. Gupta, R. Ramanath, J. Shi, and S. S. Keerthi, “Adam vs. SGD: Closing the generalization gap on image classification,” OPT2021 13th Annu. Work. Optim. Mach. Learn., pp. 1–7, 2021.
Y. Kortli, M. Jridi, A. Al Falou, and M. Atri, “Face recognition systems: A survey,” Sensors (Switzerland), vol. 20, no. 2, 2020, doi: 10.3390/s20020342.
R. Zhi, M. Liu, and D. Zhang, “A comprehensive survey on automatic facial action unit analysis,” Vis. Comput., vol. 36, no. 5, pp. 1067–1093, 2020, doi: 10.1007/s00371-019-01707-5.
J. Lamoureux and W. Luk, “An overview of low-power techniques for field-programmable gate arrays,” in Proceedings of the NASA/ESA Conference on Adaptive Hardware and Systems, AHS, 2008, pp. 338–345, doi: 10.1109/AHS.2008.71.
Raspberry pi Foundation, “Raspberry Pi 3 model B+,” 2018.
A. B. Rohan and S. Surve, “Deep learning framework for facial emotion recognition using CNN architecture,” J. Phys. Conf. Ser., vol. 2236, no. 1, pp. 1777–1784, 2022, doi: 10.1088/1742-6596/2236/1/012004.
G. K. Sahoo, S. K. Das, and P. Singh, “Deep Learning-Based Facial Emotion Recognition for Driver Healthcare,” Natl. Conf. Commun. NCC, pp. 154–159, 2022, doi: 10.1109/NCC55593.2022.9806751.
M. Jeong and B. C. Ko, “Driver’s facial expression recognition in real-time for safe driving,” Sensors (Switzerland), vol. 18, no. 12, 2018, doi: 10.3390/s18124270.
W. Liu, J. L. Qiu, W. L. Zheng, and B. L. Lu, “Comparing Recognition Performance and Robustness of Multimodal Deep Learning Models for Multimodal Emotion Recognition,” IEEE Trans. Cogn. Dev. Syst., vol. 14, no. 2, pp. 715–729, 2022, doi: 10.1109/TCDS.2021.3071170.
J. Kwon, J. Ha, D. H. Kim, J. W. Choi, and L. Kim, “Emotion Recognition Using a Glasses-Type Wearable Device via Multi-Channel Facial Responses,” IEEE Access, vol. 9, pp. 146392–146403, 2021, doi: 10.1109/ACCESS.2021.3121543.
A. Khattak, M. Z. Asghar, M. Ali, and U. Batool, “An efficient deep learning technique for facial emotion recognition,” Multimed. Tools Appl., vol. 81, no. 2, pp. 1649–1683, 2022, doi: 10.1007/s11042-021-11298-w.
I. Hina, A. Shaukat, and M. U. Akram, “Multimodal Emotion Recognition using Deep Learning Architectures,” in International Conference on Digital Futures and Transformative Technologies, ICoDT2, 2022, pp. 2–7, doi: 10.1109/ICoDT255437.2022.9787437.
A. Atanassov and D. Pilev, “Pre-trained Deep Learning Models for Facial Emotions Recognition,” 2020, doi: 10.1109/ICAI50593.2020.9311334.
T. Tumakuru, T. Tumakuru, T. Tumakuru, and T. Tumakuru, “Real Time-Employee Emotion Detection system (RtEED) using Machine Learning,” in Proceedings of the Third International Conference on Intelligent Communication Technologies and Virtual Mobile Networks, 2021, no. Icicv, pp. 759–763.
S. Jaiswal and G. C. Nandi, “Robust real-time emotion detection system using CNN architecture,” Neural Comput. Appl., vol. 32, no. 15, pp. 11253–11262, 2020, doi: 10.1007/s00521-019-04564-4.
Y. Said and M. Barr, “Human emotion recognition based on facial expressions via deep learning on high-resolution images,” Multimed. Tools Appl., vol. 80, no. 16, pp. 25241–25253, 2021, doi: 10.1007/s11042-021-10918-9.
D. Liu, Z. Wang, L. Wang, and L. Chen, “Multi-Modal Fusion Emotion Recognition Method of Speech Expression Based on Deep Learning,” Front. Neurorobot., vol. 15, no. July, pp. 1–13, 2021, doi: 10.3389/fnbot.2021.697634.
S. Miao, H. Xu, Z. Han, and Y. Zhu, “Recognizing facial expressions using a shallow convolutional neural network,” IEEE Access, vol. 7, pp. 78000–78011, 2019, doi: 10.1109/ACCESS.2019.2921220.
M. I. Georgescu, R. T. Ionescu, and M. Popescu, “Local learning with deep and handcrafted features for facial expression recognition,” IEEE Access, vol. 7, pp. 64827–64836, 2019, doi: 10.1109/ACCESS.2019.2917266.
B. S. Ajay and M. Rao, “Binary neural network based real time emotion detection on an edge computing device to detect passenger anomaly,” in Proceedings of the IEEE International Conference on VLSI Design, 2021, vol. 2021-Febru, pp. 175–180, doi: 10.1109/VLSID51830.2021.00035.
S. Saxena, S. Tripathi, and T. S. B. Sudarshan, “Deep Dive into Faces: Pose Illumination Invariant Multi-Face Emotion Recognition System,” in IEEE International Conference on Intelligent Robots and Systems, 2019, pp. 1088–1093, doi: 10.1109/IROS40897.2019.8967874.
S. M. Gowri, A. Rafeeq, and S. Devipriya, “Detection of real-time facial emotions via deep convolution neural network,” in Proceedings - 5th International Conference on Intelligent Computing and Control Systems, 2021, no. Iciccs, pp. 1033–1037, doi: 10.1109/ICICCS51141.2021.9432242.
S. Pandey, S. Handoo, and Yogesh, “Facial Emotion Recognition using Deep Learning,” in International Mobile and Embedded Technology Conference, MECON, 2022, pp. 348–352, doi: 10.1109/MECON53876.2022.9752189.
S. A. Hussain and A. Salim Abdallah Al Balushi, “A real time face emotion classification and recognition using deep learning model,” J. Phys. Conf. Ser., vol. 1432, no. 1, 2020, doi: 10.1088/1742-6596/1432/1/012087.
S. Badhe and S. Chaudhari, “Deep Learning Based Facial Emotion Recognition System,” in ITM Web of Conferences, ICACC, 2022, vol. 03058, pp. 1–5, doi: 10.1109/TIPTEKNO50054.2020.9299256.
N. Rathour et al., “Iomt based facial emotion recognition system using deep convolution neural networks,” Electron., vol. 10, no. 11, 2021, doi: 10.3390/electronics10111289.
T. Lu, S. Yeh, C. Wang, and M. Wei, “Cost-effective real-time recognition for human emotion-age-gender using deep learning with normalized facial cropping preprocess,” Multimed. Tools Appl., pp. 19845–19866, 2021.
R. Pathar, A. Adivarekar, A. Mishra, and A. Deshmukh, “Human Emotion Recognition using Convolutional Neural Network in Real Time,” 2019, doi: 10.1109/ICIICT1.2019.8741491.
M. A. Ozdemir, B. Elagoz, A. Alaybeyoglu, R. Sadighzadeh, and A. Akan, “Real time emotion recognition from facial expressions using CNN architecture,” TIPTEKNO - Tip Teknol. Kongresi, pp. 2–5, 2019, doi: 10.1109/TIPTEKNO.2019.8895215.
K.-C. Liu, C.-C. Hsu, W.-Y. Wang, and H.-H. Chiang, “Real-Time Facial Expression Recognition Based on CNN,” in International Conference on System Science and Engineering (ICSSE), 2019, no. 1, pp. 1–16.
W. Liu, J. Qiu, W. Zheng, and B. Lu, “Comparing Recognition Performance and Robustness of Multimodal Deep Learning Models for Multimodal Emotion Recognition,” IEEE Trans. Cogn. Dev. Syst., vol. 14, no. 2, pp. 715–729, 2022, doi: 10.1109/TCDS.2021.3071170.
R. Gill and J. Singh, “A Deep Learning Model for Human Emotion Recognition on Small Dataset,” in International Conference on Emerging Smart Computing and Informatics, ESCI 2022, 2022, pp. 1–5, doi: 10.1109/ESCI53509.2022.9758261.
M. A. H. Akhand, S. Roy, N. Siddique, M. A. S. Kamal, and T. Shimamura, “Facial emotion recognition using transfer learning in the deep CNN,” Electron., vol. 10, no. 9, 2021, doi: 10.3390/electronics10091036.
M. Bentoumi, M. Daoud, M. Benaouali, and A. Taleb Ahmed, “Improvement of emotion recognition from facial images using deep learning and early stopping cross validation,” Multimed. Tools Appl., vol. 81, no. 21, pp. 29887–29917, 2022, doi: 10.1007/s11042-022-12058-0.
D. Acharya, A. Billimoria, N. Srivastava, S. Goel, and A. Bhardwaj, “Emotion recognition using fourier transform and genetic programming,” Appl. Acoust., vol. 164, p. 107260, 2020, doi: 10.1016/j.apacoust.2020.107260.
A. Poulose, C. S. Reddy, J. H. Kim, and D. S. Han, “Foreground Extraction Based Facial Emotion Recognition Using Deep Learning Xception Model,” in International Conference on Ubiquitous and Future Networks, ICUFN, 2021, vol. 2021-Augus, pp. 356–360, doi: 10.1109/ICUFN49451.2021.9528706.
N. Kumari and R. Bhatia, “Efficient facial emotion recognition model using deep convolutional neural network and modified joint trilateral filter,” Soft Comput., pp. 7817–7830, 2022.
A. Vijayvergia and K. Kumar, “Selective shallow models strength integration for emotion detection using GloVe and LSTM,” Multimed. Tools Appl., pp. 28349–28363, 2021.
D. K. Jain, P. Shamsolmoali, and P. Sehdev, “Extended deep neural network for facial emotion recognition,” Pattern Recognit. Lett., vol. 120, pp. 69–74, 2019, doi: 10.1016/j.patrec.2019.01.008.
A. Agrawal and N. Mittal, “Using CNN for facial expression recognition: a study of the effects of kernel size and number of filters on accuracy,” Vis. Comput., vol. 36, no. 2, pp. 405–412, 2019, doi: 10.1007/s00371-019-01630-9.
E. Ivanova and G. Borzunov, “ScienceDirect ScienceDirect Optimization of machine learning algorithm of emotion recognition Optimization of in machine learning algorithm of emotion recognition terms of human facial expressions in terms of human facial expressions,” Procedia Comput. Sci., vol. 169, no. 2019, pp. 244–248, 2020, doi: 10.1016/j.procs.2020.02.143.
A. Gupta, S. Arunachalam, and R. Balakrishnan, “ScienceDirect ScienceDirect ScienceDirect Deep self-attention network for facial emotion recognition Deep network for facial emotion recognition Arpita self-attention,” Procedia Comput. Sci., vol. 171, no. 2019, pp. 1527–1534, 2020, doi: 10.1016/j.procs.2020.04.163.
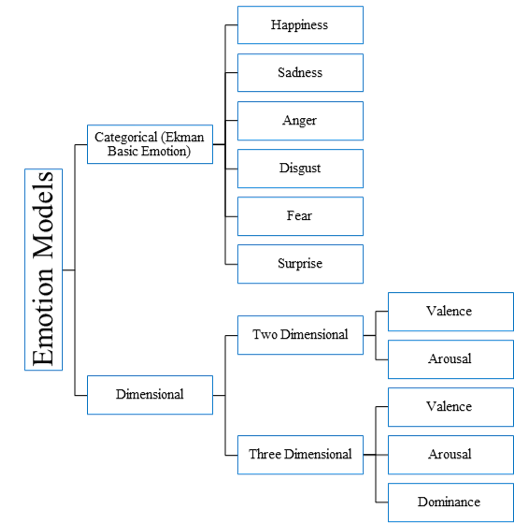
Downloads
Published
Issue
Section
License
Copyright (c) 2023 Intelligent Methods In Engineering Sciences

This work is licensed under a Creative Commons Attribution-ShareAlike 4.0 International License.