Simple AR Method for Rehabilitation Support System Based on 3D Pose Estimation
DOI:
https://doi.org/10.58190/imiens.2023.13Keywords:
rehabilitation support system, augmented reality, 3D pose estimation, deep neural network, coordinate transformation matrixAbstract
Studies have been conducted on the application of Augmented Reality to support rehabilitation of motor function recovery. The goal of these studies is to facilitate functional recovery training through patient interaction with virtual objects generated by AR. Many of them use special devices such as depth sensors to superimpose virtual objects at appropriate positions in images, but a simple method that does not require such a device is desired. In order to realize superimposition using only a personal computer (PC) with a camera, this study utilizes a deep neural network that estimates the 3-dimensional (3D) coordinates of keypoints, such as human joints, from camera images. Specifically, a coordinate transformation matrix for superimposition is calculated from the 3D coordinates of keypoints. In order to clarify the effectiveness of this method, we conducted an experiment to evaluate the superimposition accuracy. The results show that the accuracy was highest in the space near the keypoints that had been used to compute the coordinate transformation matrix, and the accuracy was even higher when the number of keypoints was small. This indicates that this method is more suitable for localized training such as hand rehabilitation than for whole-body training. Since this method can be used only with a PC with a camera, it is expected to be widely used for rehabilitation support.
Downloads
References
Y. Gu et al., “A Review of Hand Function Rehabilitation Systems Based on Hand Motion Recognition Devices and Artificial Intelligence,” Brain Science, vol. 12, 1079, 2022.
T. Hellsten, J. Karlsson, M. Shamsuzzaman, and G. Pulkkis, “The Potential of Computer Vision-based Marker-less Human Motion Analysis for Rehabilitation,” Rehabilitation Process and Outcome, vol. 10, pp. 1–12, 2021.
B. Tekin, I. Katircioglu, M. Salzmann, V. Lepetit, and P. Fu, “Structured Prediction of 3D Human Pose with Deep Neural Networks, Proc. BMVC2016,” pp. 130.1-130.11, 2016.
H. Zhou, C. Hong, Y. Han, P. Huang, and Y. Zhuang, “MH Pose: 3D Human Pose Estimation Based on High-quality Heatmap,” Proc. BIG-DATA2021, pp. 3215-3222, 2021.
S. Parajuli and M. K. Guragai, “Human Pose Estimation in 3D Using Heatmaps,” Proc. AISP2022, pp. 1-4., 2022
J. Martinez, R. Hossain, J. Romero, and J. J. Little, “A Simple yet Effective Baseline for 3D Human Pose Estimation,” Proc. ICCV2017, pp. 2640-2649, 2017.
“MediaPipePose,” [Online]. Available: https://google.github.io/mediapipe/solutions/pose. [Accessed: 10-Jan-2023].
D. Tome, C. Russell, and L. Agapito, “Lifting from the Deep: Convolutional 3D Pose Estimation from a Single Image,” Proc. CVPR2017, pp. 2500-2509, 2017.
W. Li, H. Liu, H. Tang, P. Wang, and L. V. Gool, “MHFormer: Multi-hypothesis Transformer for 3D Human Pose Estimation,” Proc. CVPR2022, pp. 13147-13156, 2022.
G. Moon, J. Y. Chang, and K. M Lee, “Camera Distance-aware Top-down Approach for 3D Multi-person Pose Estimation from a Single RGB Image,” Proc. ICCV2019, pp. 10133-10142, 2019.
Y. Cheng, B. wang, B. Y., and R. T. Tan, “Graph and Temporal Convolutional Networks for 3D Multi-person Pose Estimation in Monocular Videos,” Proc. AAAI-21, pp. 1157-1165, 2021.
L. Jin, C. Xu, X. Wang, Y. Xiao, Y. Guo, X. Nie, and J. Zhao, “Single-stage is Enough: Multi-person Absolute 3D Pose Estimation,” Proc. CVPR2022, New Orleans, USA, 13076-13085, 2022.
Y. Zhan, F. Li, R. Weng, and W. Choi, “Ray3D: Ray-based 3D Human Pose Estimation for Monocular Absolute 3D Localization,” Proc. CVPR2022, pp. 13116-13125, 2022.
H. M. Hondori, M. Khademi, L. Dodakian, S. C. Cramer, and C. V. Lopes, “A Spatial Augmented Reality Rehab System for Post-stroke Hand Rehabilitation,” Medicine Meets Virtual Reality, vol. 20, pp. 279-285, 2013.
D. Avola, L. Cinque, G. L. Foresti, and M. R. Marini, "An Interactive and Low-cost Full Body Rehabilitation Framework Based on 3D Immersive Serious Games," Journal of Biomedical Informatics, vol. 89, pp. 81–100, 2019.
Z.-R. Wang, P. Wang, L. Xing, L.-P. Mei, J. Zhao, and T. Zhang, " Leap Motion-based Virtual Reality Training for Improving Motor Functional Recovery of Upper Limbs and Neural Reorganization in Subacute Stroke Patients," Neural Regeneration Research, vol. 12(11), pp. 1823- 1831, 2017.
Y. Tokuyama, R.-P.-C. J. Rajapakse, S. Yamabe, K. Konno, and Y.-P. Hung, "A Kinect-Based Augmented Reality Game for Lower Limb Exercise," Proc. Cyberworlds2019, pp. 399- 402, 2019.
A. Latreche, R. Kelaiaia, A. Chemori, and A. Kerboua, "Reliability and Validity Analysis of MediaPipe-based Measurement System for Some Human Rehabilitation Motions," Measurement, vol. 214, 112826, 2023.
“Multimodal Motion Capture Dataset (TNT15) ,” [Online]. Available: https://www.tnt.uni-hannover.de/project/TNT15/. [Accessed: 10-Jan-2023].
C. C. Gordon et al., “Anthropometric Survey of U.S. Army Personnel: Methods and Summary Statistics,” U.S. Army Natick Soldier Research, 2014.
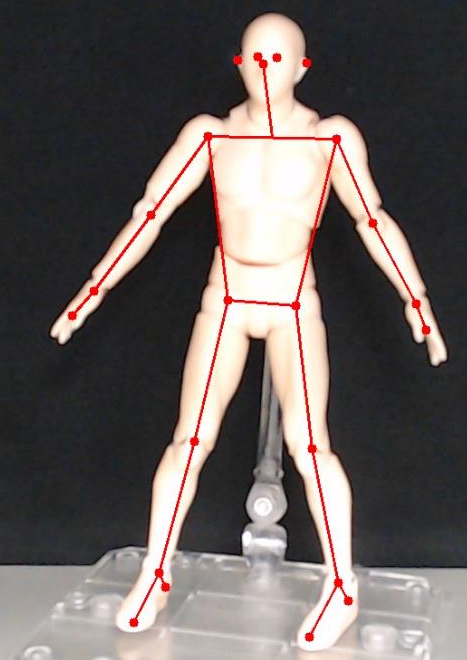
Downloads
Published
Issue
Section
License
Copyright (c) 2023 Intelligent Methods In Engineering Sciences

This work is licensed under a Creative Commons Attribution-ShareAlike 4.0 International License.