Heart Attack Risk Analysis and Estimation Using Machine Learning Methods
DOI:
https://doi.org/10.58190/imiens.2023.6Keywords:
heart disease, artificial intelligence, classification, logistic regression, analysis, visualizationAbstract
Heart disease is a disease that is difficult to diagnose and leaves serious damage to individuals like many other diseases today. It is not known whether the risk of this disease is carried or not, and it is observed that there is an increase in the number of individuals at risk today. This increase; It requires accelerating the diagnosis of the disease to humanity by making early intervention and risk analysis together with developing technologies. Machine learning methods are developing rapidly in this field, facilitating early diagnosis in medicine. Diagnosing the disease with the developed methods provides a great advantage in terms of time cost. With the developments made, the diagnosis of diseases related to more than one parameter is carried out in a very short and reliable way. In this study; with the dataset consisting of the parameters and values of carrying the risk of heart attack, the classification of the risk of heart attack with high / low probability was made using Logistic Regression, which is one of the machine learning methods. By referring to what the parameters are, the distribution and values of these parameters on the dataset are determined. Obtained values; The effect of the parameters on the result status was analyzed using visualization methods. The main purpose of these analyzes is to determine the need for corrections on the dataset before training the network. As a result of the experimental analysis, 97% overall accuracy was achieved with the proposed approach.
Downloads
References
R. S. Paffenbarger Jr, A. L. Wing, and R. T. Hyde, “Physical activity as an index of heart attack risk in college alumni,” American Journal of epidemiology, 108(3), 161-175, 1978.
R. Katarya, and S. K. Meena, “Machine learning techniques for heart disease prediction: a comparative study and analysis,” Health and Technology, 11(1), 87-97, 2021.
Bashir , Saba, et al. “Improving heart disease prediction using feature selection approaches,” In: 2019 16th international bhurban conference on applied sciences and technology (IBCAST). IEEE, 2019. p. 619-623.
A. Çalışkan, “A New Ensemble Approach for Congestive Heart Failure and Arrhythmia Classification Using Shifted One-Dimensional Local Binary Patterns with Long Short-Term Memory,” The Computer Journal, 65: 9, pp. 2535-2546, 2022.
A. Çalışkan, “Detecting human activity types from 3D posture data using deep learning models,” Biomedical Signal Processing and Control, 81, 104479, 2023.
N.Yılmaz, O.Inan and M.S. Uzer, “A new data preparation method based on clustering algorithms for diagnosis systems of heart and diabetes diseases,” Journal of medical systems, 38:48-59, 2014.
H. Kahramanli and N. Allahverdi, “Design of a hybrid system for the diabetes and heart diseases,” Expert Systems with Applications, 35.1-2:82-89, 2008.
R. Detrano, A. Janosi, and W. Steinbrunn, “International application of a new probability algorithm for the diagnosis of coronary artery disease,” American Journal of Cardiology, 64.5:304-310, 1989.
M. Gudadhe, K. Wankhade, and S. Dongre, “Decision support system for heart disease based on support vector machine and artificial neural network,” In: 2010 International Conference on Computer and Communication Technology (ICCCT). IEEE, pp. 741-745, September 2010.
E. M. Senan, I. Abunadi, M. E. Jadhav, and, S. M. Fati , “Score and Correlation Coefficient-Based Feature Selection for Predicting Heart Failure Diagnosis by Using Machine Learning Algorithms,” Computational and Mathematical Methods in Medicine, 2021.
UCI Machine Learning Repository, Heart disease data set, Available: https://archive.ics.uci.edu/ ml/datasets/Heart+Disease [Accessed: 25-Aug-2022].
R. Mogot, “Heart Attact Analysis,” Kaggle Web. (2022).
https://www.kaggle.com/code/roderikmogot/heart-attack-analysis
M. L. Vigni, C. Durante and M. Cocchi “Exploratory data analysis,” In Data Handling in Science and Technology, vol:28, pp. 55 – 126.
T. Ahmad, and M.N. Aziz, “Data preprocessing and feature selection for machine learning intrusion detection systems,” ICIC Express Lett, 13.2:, 93-101, 2019.
A. Tunc, I. Ülger, “Application of Normalization to Financial Values with Binning and Five Number Summary Methods for Feature Selection in Data Mining Applications,” Conf.: XVIII. Academic Informatics, Adnan Menderes University, 30:3, 2016.
A. Mehmood, M. Iqbal, Z. Mehmood, A. Irtaza, M. Nawaz, T. Nazir, and M. Masood “Prediction of heart disease using deep convolutional neural networks,” Arabian Journal for Science and Engineering, 46(4), 3409-3422, 2021.
J. Tolles and W. J. Meurer, “Logistic regression: relating patient characteristics to outcomes”, 316(5), pp. 533-534, 2016.
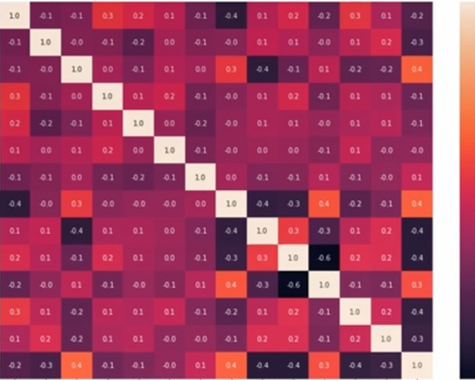
Downloads
Published
Issue
Section
License
Copyright (c) 2023 Intelligent Methods In Engineering Sciences

This work is licensed under a Creative Commons Attribution-ShareAlike 4.0 International License.