Classification of Environmental Attitudes with Artificial Intelligence Algorithms
DOI:
https://doi.org/10.58190/imiens.2024.99Keywords:
Environmental attitude, artificial Intelligence, logistic regression, support vector machine, decision tree, environmental attitude datasetAbstract
The study aims to examine people's attitudes towards the environment. Environmental education provides the necessary awareness to effectively address environmental issues. It is stated that attitudes towards the environment are very important and negative attitudes can worsen environmental problems. For this purpose, a dataset was obtained by using a scale consisting of 37 variables to a participant group consisting of 384 people. With this dataset, attitudes towards the environment have been analyzed using various classification algorithms. Logistic Regression (LR), Support Vector Machine (SVM) and Decision Tree (DT) models were used in the research. The LR, SVM, and DT models achieved 94.53%, 92.96%, and 82.55% classification success, respectively It is seen that the classification achievements of the models are at an acceptable level compared to the literature. As a result, the research sheds light on people's attitudes towards the environment through classification processes. Despite the acceptable classification achievements, alternative artificial Intelligence approaches can also be used to improve performance.
Downloads
References
Singh, R.L., Singh, P.K. (2017). Global Environmental Problems. In: Singh, R. (eds) Principles and Applications of Environmental Biotechnology for a Sustainable Future. Applied Environmental Science and Engineering for a Sustainable Future. Springer, Singapore. https://doi.org/10.1007/978-981-10-1866-4_2
Kılıç, Ç. (2018). 2-MEV (iki faktörlü çevresel değerler modeli) tutum ölçeğinin Türkçeye adaptasyonu. Yüksek Lisans Tezi, Gazi Üniversitesi Eğitim Bilimleri Enstitüsü, Ankara
Bradshaw, C. J., & Brook, B. W. (2014). Human population reduction is not a quick fix for environmental problems. Proceedings of the National Academy of Sciences, 111(46), 16610-16615. https://doi.org/10.1073/pnas.1410465111
Kaypak, Ş. (2011). Küreselleşme sürecinde sürdürülebilir bir kalkınma için sürdürülebilir bir çevre. Karamanoğlu Mehmetbey Üniversitesi Sosyal ve Ekonomik Araştırmalar Dergisi, 2011(1), 19-33.
Schlüter, M., Haider, L. J., Lade, S. J., Lindkvist, E., Martin, R., Orach, K., ... & Folke, C. (2019). Capturing emergent phenomena in social-ecological systems. Ecology and Society, 24(3). https://www.jstor.org/stable/26796977
Doğan, F., & Keleş, Y. (2020). Environmental awareness and environmental behavior in secondary and high school students. NEÜ Ereğli Eğitim Fakültesi Dergisi, 2(1), 80-90.
Vaughan, C., Gack, J., Solorazano, H. & Ray, R. (2003). The effect on environmental education on schoolchildren, their parents, and community members: a study of intergenerational and intercommunity learning. The Journal of Environmental Education. 34(3), 12-21 https://doi.org/10.1080/00958960309603489
Esteban Ibáñez M, Musitu Ferrer D, Amador Muñoz LV, Claros FM, Olmedo Ruiz FJ. University as Change Manager of Attitudes towards Environment (The Importance of Environmental Education). Sustainability. 2020; 12(11):4568. https://doi.org/10.3390/su12114568
Öztürk, S., Tekin, N. & Yılmaz, S. (2023). The Opinions of Eighth Grade Students on Renewable Energy Sources in their Environment: A Local Perspective. Ahmet Keleşoğlu Eğitim Fakültesi Dergisi (AKEF) Dergisi, 5(2), 474-487. https://doi.org/10.38151/akef.65
Nurwidodo, N., Amin, M., Ibrohim, I., & Sueb, S. (2020). The role of eco-school program (adiwiyata) towards environmental literacy of high school students. European Journal of Educational Research, 9(3), 1089-1103. https://doi.org/10.12973/eu-jer.9.3.1089
Uralovich, K. S., Toshmamatovich, T. U., Kubayevich, K. F., Sapaev, I., Saylaubaevna, S. S., Beknazarova, Z., & Khurramov, A. (2023). A primary factor in sustainable development and environmental sustainability is environmental education. Caspian Journal of Environmental Sciences, 21(4), 965-975. doi: 10.22124/cjes.2023.7155
Stevenson, R. B. (2007). Schooling and environmental education: contradictions in purpose and practice. Environmental Education Research, 13(2), 139–153. https://doi.org/10.1080/13504620701295726
Greenwald, A. G., & Banaji, M. R. (1995). Implicit social cognition: Attitudes, self-esteem, and stereotypes. Psychological Review, 102(1), 4–27. https://doi.org/10.1037/0033-295X.102.1.4
Oskamp, S., & Schultz, P.W. (2005). Attitudes and Opinions (3rd ed.). Psychology Press. https://doi.org/10.4324/9781410611963
Uzun, N. ve Sağlam, N. (2006). “Ortaöğretim Öğrencileri İçin Çevresel Tutum Ölçeği Geliştirme ve Geçerliliği.” Hacettepe Üniversitesi Eğitim Fakültesi Dergisi, 30, 240-250.
Akçay, S. & Şengül, H. (2023). A Study on Environmental Literacy of Middle School Students. Ahmet Keleşoğlu Eğitim Fakültesi Dergisi (AKEF) Dergisi, 5(1), 139-169. https://doi.org/10.38151/akef.2023.48
Rickinson, M. (2001). Learners and Learning in Environmental Education: A critical review of the evidence. Environmental Education Research, 7(3), 207–320. https://doi.org/10.1080/13504620120065230
Erten, S. (2005). Okul öncesi öğretmen adaylarında çevre dostu davranışların araştırılması. Hacettepe Üniversitesi Eğitim Fakültesi Dergisi, 28, 91-100
Ajzen, I., Fishbein, M., Lohmann, S., & Albarracín, D. (2018). The influence of attitudes on behavior. The handbook of attitudes, volume 1: Basic principles, 197-255. https://doi.org/10.4324/9781315178103
Sukma, E., Ramadhan, S., & Indriyani, V. (2020, March). Integration of environmental education in elementary schools. In Journal of Physics: Conference Series (Vol. 1481, No. 1, p. 012136). IOP Publishing. https://doi.org/10.1088/1742-6596/1481/1/012136
Bergman, B. G. (2015). Assessing impacts of locally designed environmental education projects on students’ environmental attitudes, awareness, and intention to act. Environmental Education Research, 22(4), 480–503. https://doi.org/10.1080/13504622.2014.999225
Athman, J. A., & Monroe, M. C. (2001). Elements of Effective Environmental Education Programs.
Aydoğan, Y., Okyay, Ö., & Aksut, P. How to Raise the Environmental Awareness in Children. Environment and Ecology at the Beginning of 21st Century, 700.
Palmer, J. (2002). Environmental education in the 21st century: Theory, practice, progress and promise. Routledge.
Yadav, S. K., Banerjee, A., Jhariya, M. K., Meena, R. S., Raj, A., Khan, N., ... & Sheoran, S. (2022). Environmental education for sustainable development. In Natural Resources Conservation and Advances for Sustainability (pp. 415-431). Elsevier. https://doi.org/10.1016/B978-0-12-822976-7.00010-7
Taspinar, Y. S., Cinar, I., & Koklu, M. (2021). Prediction of computer type using benchmark scores of hardware units. Selcuk University Journal of Engineering Sciences, 20(1), 11-17.
Vapnik, V. (1999). The nature of statistical learning theory. Springer science & business media. https://doi.org/10.1007/978-1-4757-3264-1
Powers, D. M. (2020). Evaluation: from precision, recall and F-measure to ROC, informedness, markedness and correlation. arXiv preprint arXiv:2010.16061. https://doi.org/10.48550/arXiv.2010.16061
Sun, A., & Lim, E.-P. (2001). Hierarchical text classification and evaluation. Proceedings 2001 IEEE International Conference on Data Mining, https://doi.org/10.1109/ICDM.2001.989560
Tang, T. A., Mhamdi, L., McLernon, D., Zaidi, S. A. R., & Ghogho, M. (2018). Deep recurrent neural network for intrusion detection in sdn-based networks. 2018 4th IEEE Conference on Network Softwarization and Workshops (NetSoft), https://doi.org/10.1109/NETSOFT.2018.8460090
Sokolova, M., Japkowicz, N., Szpakowicz, S. (2006). Beyond Accuracy, F-Score and ROC: A Family of Discriminant Measures for Performance Evaluation. In: Sattar, A., Kang, Bh. (eds) AI 2006: Advances in Artificial Intelligence. AI 2006. Lecture Notes in Computer Science(), vol 4304. Springer, Berlin, Heidelberg. https://doi.org/10.1007/11941439_114
Ferri, C., Hernández-Orallo, J., & Modroiu, R. (2009). An experimental comparison of performance measures for classification. Pattern recognition letters, 30(1), 27-38. https://doi.org/10.1016/j.patrec.2008.08.010
Dwivedi, A. K. (2018). Performance evaluation of different machine learning techniques for prediction of heart disease. Neural Computing and Applications, 29, 685-693. https://doi.org/10.1007/s00521-016-2604-1
Browne, M. W. (2000). Cross-validation methods. Journal of mathematical psychology, 44(1), 108-132. https://doi.org/10.1006/jmps.1999.1279
Anguita, D., Ghelardoni, L., Ghio, A., Oneto, L., & Ridella, S. (2012). The'K'in K-fold Cross Validation. ESANN,
Berrar, D. (2019). Cross-Validation. . https://doi.org/10.1016/B978-0-12-809633-8.20349-X
Kohavi, R., & John, G. H. (1997). Wrappers for feature subset selection. Artificial intelligence, 97(1-2), 273-324. https://doi.org/10.1016/S0004-3702(97)00043-X
Shao, J. (1993). Linear Model Selection by Cross-validation. Journal of the American Statistical Association, 88(422), 486–494. https://doi.org/10.1080/01621459.1993.10476299
Raschka, S. (2018). Model evaluation, model selection, and algorithm selection in machine learning. arXiv preprint arXiv:1811.12808. https://doi.org/10.48550/arXiv.1811.12808
Ramezan C, A. Warner T, E. Maxwell A. Evaluation of Sampling and Cross-Validation Tuning Strategies for Regional-Scale Machine Learning Classification. Remote Sensing. 2019; 11(2):185. https://doi.org/10.3390/rs11020185
Frank, E., Wang, Y., Inglis, S., Holmes, G., & Witten, I. H. (1998). Using model trees for classification. Machine learning, 32, 63-76. https://doi.org/10.1023/A:1007421302149
Landwehr, N., Hall, M., & Frank, E. (2005). Logistic model trees. Machine learning, 59, 161-205. https://doi.org/10.1007/s10994-005-0466-3
Sharma, P. (2014). Comparative analysis of various decision tree classification algorithms using WEKA. International Journal on Recent and Innovation Trends in Computing and Communication, 3(2), 684-690.
Chan, K.-Y., & Loh, W.-Y. (2004). LOTUS: An algorithm for building accurate and comprehensible logistic regression trees. Journal of Computational and Graphical Statistics, 13(4), 826-852. https://doi.org/10.1198/106186004X13064
Friedman, J., Hastie, T., & Tibshirani, R. (2000). Additive logistic regression: a statistical view of boosting (with discussion and a rejoinder by the authors). The annals of statistics, 28(2), 337-407. https://doi.org/10.1214/aos/1016218223
Cristianini, N., & Shawe-Taylor, J. (2000). An introduction to support vector machines and other kernel-based learning methods. Cambridge university press.
Cervantes, J., Garcia-Lamont, F., Rodríguez-Mazahua, L., & Lopez, A. (2020). A comprehensive survey on support vector machine classification: Applications, challenges and trends. Neurocomputing, 408, 189-215. https://doi.org/10.1016/j.neucom.2019.10.118
Pavlidis, P., Wapinski, I., & Noble, W. S. (2004). Support vector machine classification on the web. Bioinformatics, 20(4), 586-587. https://doi.org/10.1093/bioinformatics/btg461
Platt, J. (1998). Sequential minimal optimization: A fast algorithm for training support vector machines.
Murthy, S. K. (1998). Automatic construction of decision trees from data: A multi-disciplinary survey. Data mining and knowledge discovery, 2, 345-389. https://doi.org/10.1023/A:1009744630224
Pal, M., & Mather, P. M. (2003). An assessment of the effectiveness of decision tree methods for land cover classification. Remote sensing of environment, 86(4), 554-565. https://doi.org/10.1016/S0034-4257(03)00132-9
Tu, M. C., Shin, D., & Shin, D. (2009). A comparative study of medical data classification methods based on decision tree and bagging algorithms. 2009 Eighth IEEE International Conference on Dependable, Autonomic and Secure Computing, https://doi.org/10.1109/DASC.2009.40
Bansal, M., Goyal, A., & Choudhary, A. (2022). A comparative analysis of K-nearest neighbor, genetic, support vector machine, decision tree, and long short term memory algorithms in machine learning. Decision Analytics Journal, 3, 100071. https://doi.org/10.1016/j.dajour.2022.100071
Aitkenhead, M. J. (2008). A co-evolving decision tree classification method. Expert Systems with Applications, 34(1), 18-25. https://doi.org/10.1016/j.eswa.2006.08.00
Kotsiantis, S. B. (2013). Decision trees: a recent overview. Artificial Intelligence Review, 39, 261-283. https://doi.org/10.1007/s10462-011-9272-4
Yadav, S. K., & Pal, S. (2012). Data mining: A prediction for performance improvement of engineering students using classification. arXiv preprint arXiv:1203.3832. https://doi.org/10.48550/arXiv.1203.3832
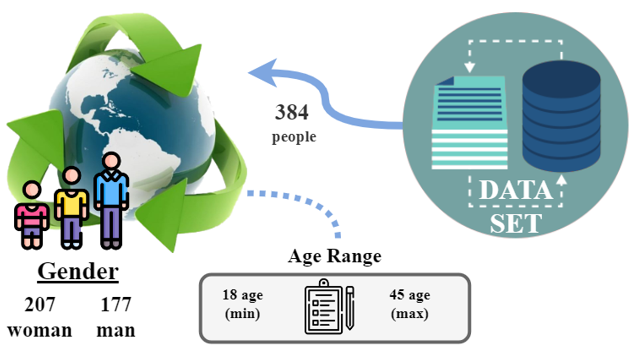
Published
Issue
Section
License
Copyright (c) 2024 Intelligent Methods In Engineering Sciences

This work is licensed under a Creative Commons Attribution-ShareAlike 4.0 International License.